Documenting My Assumptions on AI and Schol Comms
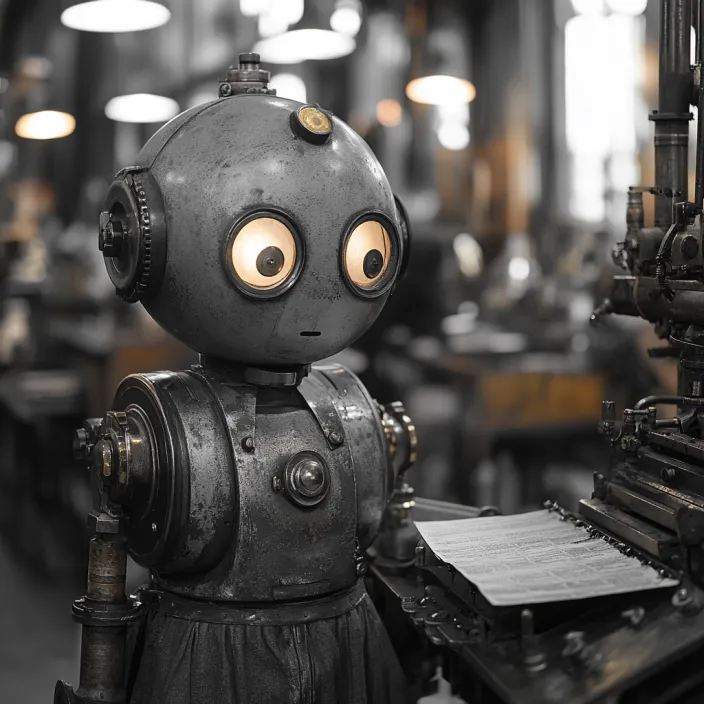
And Why I’m Already Planning for the Next Leap
AI is moving fast—ridiculously fast. Keeping a clear view of where it’s heading requires staying up to date with developments and continuously assessing how they shape my next steps.
More than ever, time is a critical factor. I find myself thinking in two timeframes:
- Scenario A: The short-term (1–2 years), where AI is already reshaping workflows.
- Scenario B: The longer-term, where AI’s impact is still coming into focus.
So far, I can only think out the next one to two years—things are changing too quickly to see much further. But the outlines of a deeper transformation are beginning to emerge.
Thinking in a 1–2 year window, however, is useful because it limits the number of variables we need to consider. I don’t believe AI will fundamentally eliminate the need for publishing or researchers within this timeframe—though in 40 years, that might be a different story.
For now, we can assume that many environmental factors will remain stable, allowing us to focus on near-term transformations rather than existential disruptions. We are, in effect, in an age of transition—a segue between traditional models and AI-driven workflows.
Why Write These Assumptions Down Now?
When everything moves this fast, it’s easy to lose track of just how much has changed. Writing these thoughts down now helps in three ways:
- Setting a baseline. In the coming months and years, I can look back and see what held up, what changed, and what I got completely wrong.
- Encouraging discussion. Others might see the same trends—or spot things I’ve overlooked.
- Staying adaptable. Revisiting and updating my thinking regularly prevents me from clinging to outdated assumptions.
Even if some of these predictions don’t unfold exactly as expected, writing them down helps bring clarity to the chaos. There are many underlying assumptions to consider and even more consequences to explore. For example, what does all of this mean for open source as a movement? It’s a question worth deeper reflection—one I’ll likely explore in a future piece.
For now, though, three key developments stand out as shaping the next 1–2 years:
- The rapid improvement of AI coding capabilities.
- The rise of collaborative user agents (CUA).
- The evolution of AI agents into true digital workers.
Here’s how I see these three developments unfolding and how it effects what I do - build publishing platforms - over the next 1 - 2 years.
AI and Coding: Small Teams, Big Impact
At a recent event, OpenAI’s Sam Altman claimed their next-gen model, currently being tested under the codename o3, can perform at the level of the 50th-best software developer in the world on certain tasks.
Whether that exact ranking is accurate is debatable - it seems ranking programmers is based on testing various asks, not on the ability to build a complete platform.
But the bigger takeaway is clear: AI is advancing at a pace that traditional software development has never seen before.
This shift has major implications:
- AI-powered coding assistants are already making software development dramatically more efficient.
- As AI improves, a small team—or even a single developer—will be able to build at a scale that previously required much larger teams.
- The advantage shifts toward nimble teams that move fast and leverage AI effectively.
I believe development teams will build software faster than ever, thanks to AI co-pilots that assist with coding, testing, and project management. Tasks that once required large engineering teams—writing boilerplate code, debugging, optimizing performance, and even managing workflows—will be streamlined by AI.
This levels the playing field. Small, agile organizations like Kotahi will be able to rival the entrenched workforce of large enterprise systems. With AI reducing the overhead of software development, innovation will no longer be constrained by team size. Instead, success will depend on vision, execution, and the ability to integrate AI-driven development tools effectively.
Another key impact is faster deployment cycles. In Kotahi, we’re moving toward daily releases, leveraging AI-assisted development to roll out features, bug fixes, and optimizations in real time. This dramatically improves responsiveness to user needs.
Now, compare that to incumbent systems, where iteration cycles are measured in quarters or even years. As AI-driven teams accelerate development, these legacy platforms will start to look like the dinosaurs they are—too slow to adapt, too rigid to compete.
The result? Faster iteration, more experimentation, and a world where small teams can disrupt markets that were once dominated by large incumbents.
Computer-Using Agents (CUA): AI as a Digital Worker
One of the biggest shifts in AI isn’t just about better models—it’s about AI’s ability to interact with existing software interfaces. Instead of simply answering questions or generating text, AI is beginning to handle actual digital workflows.
OpenAI has introduced Operator, an AI agent designed to interact with software just like a human user. If this capability improves in reliability and speed, it removes the need for backend modifications—AI can simply use the same software interfaces humans use. That unlocks huge potential for automation, streamlining workflows, and reducing manual, repetitive tasks.
This has two immediate implications for my world:
- Software interfaces will evolve for AI collaboration.
Instead of treating AI as a magic tool that replaces jobs, interfaces will need to support collaborative AI use. Right now, software is built only for human users. As AI takes on more tasks, interfaces must facilitate smooth interactions where humans can oversee, refine, and improve AI-driven outcomes. Kotahi is pretty much developed with collaboration in mind, so its actually not too great a difference although some interactions and functions require deeper rethinking. - AI could eliminate the pain of switching software platforms.
Data migration is one of the biggest barriers to adopting new tools in publishing. Moving from one system to another usually requires custom scripts, manual oversight, and significant frustration. If AI agents can operate software interfaces like a human, switching platforms could be as logging into both systems to give them admin access to all your records and then saying: "Transfer everything from System A to System B."
The friction of transitioning to better tools could disappear in a year or two.
AI Agents: Automating Complex Functions
AI agents are becoming far more sophisticated. For example, Deep Research from OpenAI is designed to handle complex tasks by breaking them into multiple steps and systematically processing them to return a well-reasoned outcome.
This marks a shift from AI assisting with tasks to AI managing specific parts of a workflow.
This could be similar to a strategy for start ups already seen in Silicon valley around spreadsheets. Some companies in Silicon Valley have followed a simple playbook:
- Find a process people are handling manually (eg. in spreadsheets).
- Build software that does that task and makes it more efficient.
Now, a new model is emerging—one driven by AI. Instead of just replacing spreadsheets, it involves:
- Identifying the core functions within an industry.
- Build AI agents that focus on one task and makes it more efficient.
- Automating the high-value ones first.
- Iterate until the entire workflow is covered.
Looking at scholarly communications in the next years there are a number of low hanging fruit:
- Manuscript Preparation
- Formatting manuscripts to journal-specific styles
- Pre-flight compliance checks before submission
- Reference & Citation Management
- Automated citation formatting
- Cross-checking references against databases
- Metadata Generation
- Automatic extraction and enrichment
- Standardized indexing for DOIs, ORCID, and repositories
- Compliance & Ethical Checks
- Funder and institutional policy compliance
- Conflict-of-interest detection
- Peer Review Facilitation
- AI-assisted reviewer matching
- Automated summarization of reviewer feedback
- Language & Readability Enhancement
- Automated grammar and clarity improvements
- AI-assisted translation
- Production & Multi-Format Publishing
- Automated typesetting for print-ready PDFs
- Dynamic conversion to XML, EPUB, and HTML
- Post-Publication Monitoring
- AI-powered impact tracking
- Automated alerts for errata and retractions
A Glimpse Into the Next Year
If I had to predict where we’ll be in 12–18 months:
✅ Development teams will build software faster than ever, enabling small teams to challenge giants.
✅ Migrations will be easier, as AI agents take over the tedious work of moving data across platforms. Enabling publishers to move form their legacy systems to new systems a lot more cheaply, painlessly, and quicker.
✅ Publishing workflow steps will necessary be designed to be collaborative (to support human-computer collaboration) and each step will increasingly be automated.
Why It Matters
This isn’t just about playing with new tech. These advancements change the cost, speed, and scale of what’s possible in software development, publishing, and business operations.
For the software platform space in scholarly comms, the implications are huge:
- The cost of building software is dropping. Legacy players in the scholarly communications market, heavily invested in large development teams, will find themselves overextended and forced to scale down. Meanwhile, smaller organizations can compete on both feature development and speed, outpacing established players in innovation.
- The time cost and pain required to switch tools is shrinking. Enabling existing customers to exit expensive legacy technologies.
- Small, AI-powered teams can now challenge larger incumbents with more efficient, faster moving, and lower-cost solutions.
I’ll revisit these assumptions periodically and adjust accordingly.
Member discussion